[ad_1]
The vital to building adaptable machine-finding out models that are able of reasoning like men and women do may possibly not be feeding them oodles of education info. Alternatively, a new study indicates, it may well arrive down to how they are educated. These results could be a big move towards improved, much less mistake-prone synthetic intelligence products and could support illuminate the secrets and techniques of how AI systems—and humans—learn.
Individuals are master remixers. When men and women recognize the relationships amongst a set of factors, this sort of as food elements, we can blend them into all types of tasty recipes. With language, we can decipher sentences we have never encountered ahead of and compose complicated, initial responses because we grasp the underlying meanings of text and the guidelines of grammar. In complex conditions, these two illustrations are proof of “compositionality,” or “systematic generalization”—often viewed as a key principle of human cognition. “I assume that is the most crucial definition of intelligence,” claims Paul Smolensky, a cognitive scientist at Johns Hopkins College. “You can go from realizing about the parts to working with the whole.”
Real compositionality may be central to the human head, but device-finding out builders have struggled for many years to verify that AI methods can achieve it. A 35-12 months-old argument made by the late philosophers and cognitive researchers Jerry Fodor and Zenon Pylyshyn posits that the theory may possibly be out of access for regular neural networks. Today’s generative AI models can mimic compositionality, generating humanlike responses to created prompts. Nonetheless even the most advanced versions, such as OpenAI’s GPT-3 and GPT-4, continue to drop brief of some benchmarks of this skill. For instance, if you question ChatGPT a concern, it may possibly to begin with deliver the accurate response. If you go on to deliver it adhere to-up queries, on the other hand, it could fail to stay on subject matter or start contradicting by itself. This implies that although the styles can regurgitate information and facts from their education data, they don’t truly grasp the this means and intention driving the sentences they create.
But a novel schooling protocol that is focused on shaping how neural networks find out can enhance an AI model’s potential to interpret information and facts the way human beings do, in accordance to a study posted on Wednesday in Mother nature. The conclusions counsel that a sure method to AI training may generate compositional equipment studying models that can generalize just as properly as people—at minimum in some occasions.
“This investigation breaks significant ground,” states Smolensky, who was not included in the examine. “It accomplishes anything that we have wanted to execute and have not previously succeeded in.”
To train a procedure that appears capable of recombining parts and knowledge the indicating of novel, elaborate expressions, researchers did not have to make an AI from scratch. “We didn’t will need to essentially improve the architecture,” states Brenden Lake, guide author of the analyze and a computational cognitive scientist at New York University. “We just experienced to give it follow.” The scientists began with a regular transformer model—a product that was the exact form of AI scaffolding that supports ChatGPT and Google’s Bard but lacked any prior text training. They ran that simple neural network through a specifically built set of tasks meant to instruct the method how to interpret a designed-up language.
The language consisted of nonsense text (these kinds of as “dax,” “lug,” “kiki,” “fep” and “blicket”) that “translated” into sets of vibrant dots. Some of these invented terms have been symbolic phrases that right represented dots of a certain coloration, even though other folks signified functions that changed the purchase or variety of dot outputs. For occasion, dax represented a uncomplicated crimson dot, but fep was a purpose that, when paired with dax or any other symbolic word, multiplied its corresponding dot output by three. So “dax fep” would translate into 3 pink dots. The AI schooling bundled none of that info, however: the scientists just fed the design a handful of examples of nonsense sentences paired with the corresponding sets of dots.
From there, the review authors prompted the design to make its have series of dots in response to new phrases, and they graded the AI on no matter if it experienced appropriately adopted the language’s implied rules. Before long the neural network was capable to reply coherently, pursuing the logic of the nonsense language, even when launched to new configurations of text. This suggests it could “understand” the built-up policies of the language and utilize them to phrases it hadn’t been experienced on.
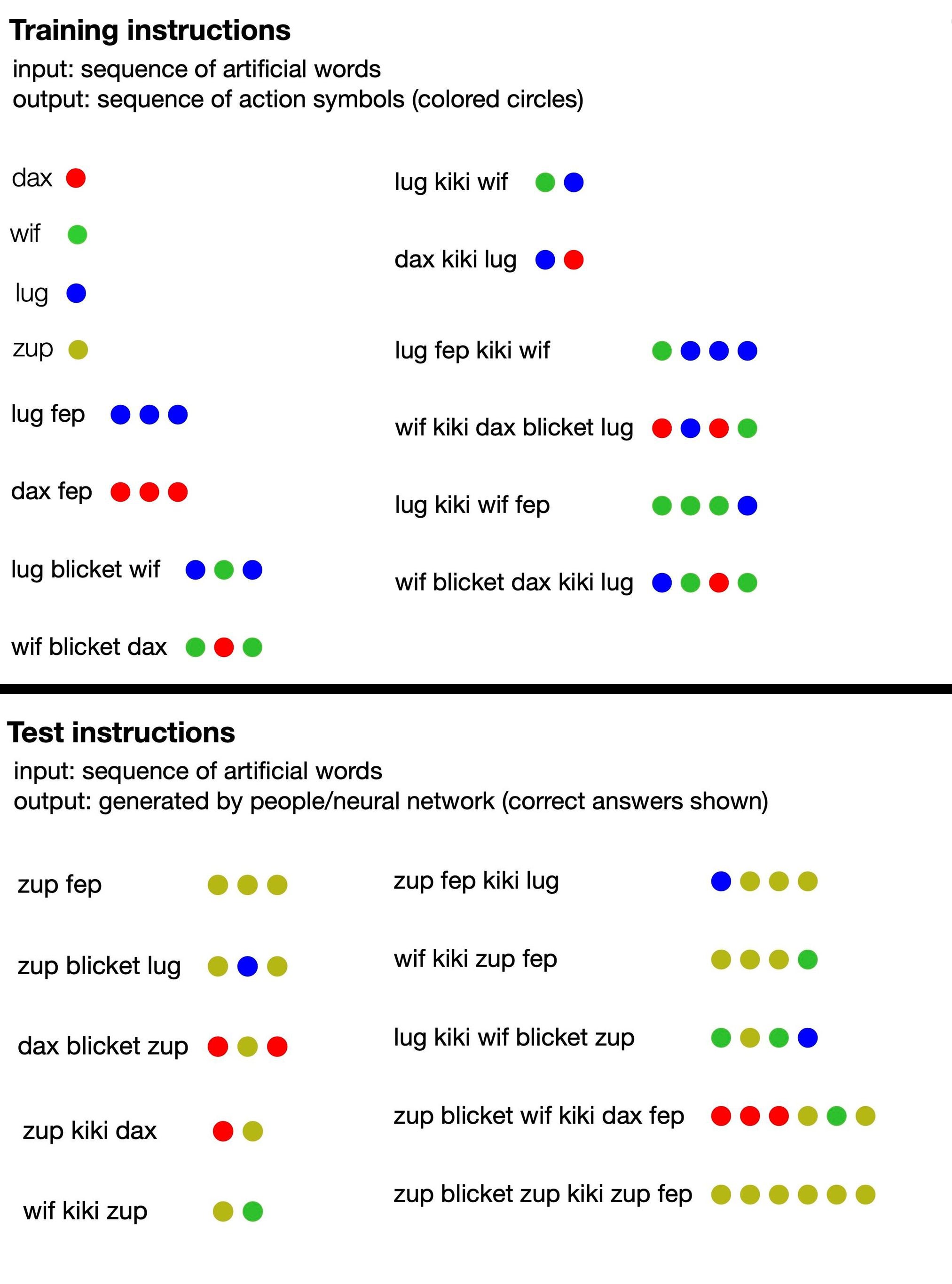
In addition, the researchers analyzed their educated AI model’s being familiar with of the created-up language versus 25 human individuals. They observed that, at its best, their optimized neural network responded 100 per cent properly, when human responses have been suitable about 81 percent of the time. (When the workforce fed GPT-4 the teaching prompts for the language and then asked it the test questions, the massive language product was only 58 p.c correct.) Specified supplemental coaching, the researchers’ typical transformer model commenced to mimic human reasoning so very well that it produced the similar mistakes: For occasion, human members generally erred by assuming there was a 1-to-1 connection amongst particular terms and dots, even even though a lot of of the phrases didn’t observe that pattern. When the design was fed illustrations of this actions, it quickly commenced to replicate it and built the error with the exact frequency as human beings did.
The model’s general performance is particularly amazing, specified its smaller measurement. “This is not a substantial language design experienced on the entire Online this is a somewhat compact transformer skilled for these jobs,” says Armando Photo voltaic-Lezama, a personal computer scientist at the Massachusetts Institute of Engineering, who was not included in the new examine. “It was intriguing to see that nevertheless it’s ready to show these kinds of generalizations.” The locating implies that in its place of just shoving ever more coaching information into machine-understanding types, a complementary approach could be to supply AI algorithms the equal of a concentrated linguistics or algebra class.
Photo voltaic-Lezama claims this instruction strategy could theoretically supply an alternate route to greater AI. “Once you have fed a design the entire Web, there’s no second Online to feed it to additional improve. So I believe techniques that power products to purpose better, even in synthetic tasks, could have an influence going forward,” he says—with the caveat that there could be difficulties to scaling up the new coaching protocol. Simultaneously, Photo voltaic-Lezama thinks this sort of studies of smaller styles assist us better understand the “black box” of neural networks and could lose light on the so-named emergent skills of larger AI programs.
Smolensky adds that this review, along with similar get the job done in the upcoming, could possibly also boost humans’ knowledge of our individual thoughts. That could assistance us layout methods that limit our species’ mistake-susceptible tendencies.
In the existing, nonetheless, these positive aspects stay hypothetical—and there are a few of huge restrictions. “Despite its successes, their algorithm doesn’t address just about every problem lifted,” claims Ruslan Salakhutdinov, a pc scientist at Carnegie Mellon College, who was not involved in the review. “It doesn’t instantly manage unpracticed sorts of generalization.” In other text, the coaching protocol served the model excel in a single variety of process: learning the designs in a bogus language. But provided a total new undertaking, it couldn’t use the similar ability. This was evident in benchmark exams, in which the product failed to take care of for a longer time sequences and couldn’t grasp beforehand unintroduced “words.”
And crucially, just about every pro Scientific American spoke with mentioned that a neural network capable of constrained generalization is incredibly different from the holy grail of artificial common intelligence, wherein computer system types surpass human potential in most jobs. You could argue that “it’s a incredibly, incredibly, extremely small stage in that course,” Solar-Lezama claims. “But we’re not conversing about an AI getting capabilities by itself.”
From constrained interactions with AI chatbots, which can present an illusion of hypercompetency, and abundant circulating hoopla, quite a few folks may possibly have inflated suggestions of neural networks’ powers. “Some men and women may find it astonishing that these sorts of linguistic generalization tasks are definitely difficult for devices like GPT-4 to do out of the box,” Photo voltaic-Lezama claims. The new study’s conclusions, even though interesting, could inadvertently serve as a truth check out. “It’s really vital to hold keep track of of what these devices are able of carrying out,” he states, “but also of what they cannot.”
[ad_2]
Resource backlink